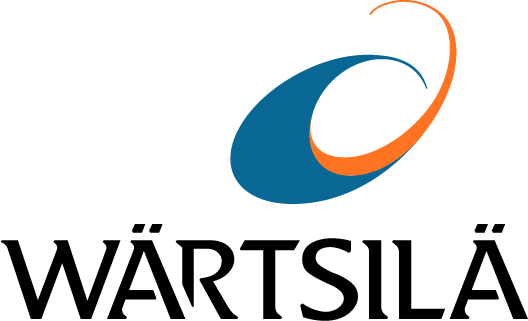
Artificial Intelligence (AI) seems to be everywhere.
AI is integral to the way some of the largest and most successful companies, such as Amazon, Google and Uber, run their businesses.
It is putting innovative, headline-grabbing products and services on the market, such as driverless cars, applications that can have coherent text-based conversations with humans, and a computer programme that defeated a professional player of the Chinese board game Go 5 times out of 5.
However, all these capabilities came at a huge financial cost.
Technology giants have paid billions to acquire AI start-ups and absorb their capabilities. One of the largest and most capable AI language models, Microsoft, and Nvidia’s Megatron-Turing Natural Language Generation (MT-NLG), cost millions of dollars to create and the Google subsidiary DeepMind is estimated to have spent USD 35 million training the programme that won at Go. Further, Tesla is designing its own computer chips just to train AI models to drive autonomously.
Such eyewatering investments come with a pronounced downside. “If only large companies with access to capital and reach are able to invest in AI, innovation will undoubtedly be slowed down,” says Ori Goshen, co-founder and co-CEO of the AI lab and product company, AI21 Labs. “The research arms of large corporations are usually managed as separate entities, such as Google Brain and Facebook Artificial Intelligence Research (FAIR), which distance them from real-world use cases and commercial applications.”
Besides its much-discussed capabilities, companies are increasingly using AI in their everyday operations to increase efficiency, anticipate and better fulfil customer expectations and stay competitive.
“AI could potentially deliver additional economic output of around USD 13 trillion by 2030, boosting global GDP by about 1.2 per cent a year,” a McKinsey Global Institute report states. A study by PricewaterhouseCoopers (PwC) estimates that “global GDP may increase by up to 14 % (the equivalent of USD 15.7 trillion) by 2030 as a result of the accelerating development and take-up of AI.”
For AI to live up to its huge potential, the ecosystem needs to look at the smaller players more closely.
Deep tech start-ups that challenge the incumbent are necessary to push the field of AI forward.
“Smaller organisations benefit from two key advantages: speed and agility. It's the nature of the beast: start-ups either innovate fast and find an efficient, quick way to grab market share, or die. Deep tech start-ups that challenge the incumbent are necessary to push the field of AI forward,” says Goshen.
Reducing the cost of AI research and innovation, therefore, becomes crucial.
One possible solution to reduce costs is ‘neural network pruning’. As the name suggests, the size of the AI model is reduced, or ‘pruned’, while maintaining its accuracy and performance. This results in a model that retains its architecture while having fewer parameters to deal with, thus reducing the computational needs of the system.
However, the most promise lies in changing the approach to training AI models.
Existing AI models typically take a software-centric approach, where the data is fixed, and programmes are built by software and AI experts to learn from that data. This results in very large datasets which are expensive to work with and often contain superfluous data.
Moving towards a data-centric approach, where the focus is on obtaining high-quality data that covers all the cases and variations needed to train models, results in smaller datasets and shorter implementation timelines, thus reducing overall AI project costs.
The biggest advantage, however, is that this approach utilises the company’s domain experts, not just software and AI experts, to contribute to the building and training of AI models, making them more accurate and increasing performance when it comes to business requirements.
As societies and economies around the world increasingly rely on technological solutions to a myriad of problems, AI-led innovation holds immeasurable promise. However, the entire ecosystem, as well as stakeholders and allied technologies, needs to recognise the very real barriers that exist.
“The industry is beginning to realise that increasing the size of AI models leads to diminishing returns, meaning serving costs become unsustainable economically. Thus, new architectures are called for, and the small players in the market are exactly the type of organisations that will lead the way, simply because they must,” Goshen concludes.
If AI-led innovation is to live up to its potential, it needs to become more equitable, democratised, and sustainable.