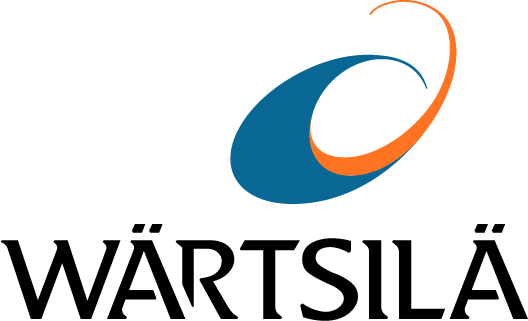
As global enterprises rely more heavily on artificial intelligence (AI) to address their more complex needs, we are seeing an all-time low in female students and professionals across all levels of the ICT, STEM (science, technology, engineering, and mathematics) and AI sectors.
In fact, a 2020 World Economic Forum report found that just over a quarter of AI professionals globally were women. Similarly, the Kapor Center in California reported that as few as 13% of women working in tech identified as Black, Latinx or Native American – with only 8% identifying as non-binary.
With this large-scale exclusion comes staggering setbacks: from lower job opportunities to reduced physical safety due to security systems failing to identify women as accurately as men. In a male-dominated digital world, have half the population’s needs taken a back seat?
“Representation on all levels – if we don’t take accountability for greater representation, AI can become counterproductive and reinforce the gender gap,” begins Kejsi Gjordeni, former Strategy and Operations Manager at Visma, a company that provides business software and IT related development and consultancy.
“We as humans are biased. Studies show that more diverse teams are performing better. It’s a no-brainer that we should have more diversity in all senses,” adds Gjordeni.
A breeding ground for reinforcing existing biases is easily propagated when most AI developers worldwide are male. Technology designed and built almost exclusively by men can become skewed to represent their individual experiences.
“Some regulations, such as the ethics of AI, have been put in place but we need to be stricter in reviewing the data that we work on, and checking that all groups are well represented in our datasets,” says Arjeta Peshtani, a former Machine Learning Engineer at Wärtsilä.
For example, Amazon had to pull an AI-powered tool used for recruitment purposes in 2018 when male candidates were recommended for job roles at a disproportionately higher rate than their female counterparts. The machine learning system used was taught to review applications submitted over 10 years. Since men held most tech positions over the set period, the algorithm taught itself to discredit any application which included the word ‘women’.
Representation on all levels – if we don’t take accountability for greater representation, AI can become counterproductive and reinforce the gender gap.
Over a third of companies now employ AI to leverage their operational capabilities, according to the 2022 IBM Global AI Adoption Index. The report describes how one in four businesses has turned to machine learning to address comprehensive issues ranging from employment shortages to environmental, social, and governance-related ambitions.
Implementing AI in our daily lives allows for countless systems and operations to be automated. With this comes the promising potential to enhance and redefine all areas of our society. However, Peshtani notes that work within a machine learning model doesn’t end by putting it into production.
“Another essential part is to monitor its performance. Data that is used for these models might change over time and the data scientist needs to be aware of these changes,” says Peshtani.
Methodology for optimising machine learning models might also serve as a partial solution to help bridge the AI gender gap. It is crucial to bring more young girls and women into the field and to monitor, retain, and develop their overarching educational and career paths.
While Gjordeni’s career progressed naturally from a technical role to a more strategy-based position due to her personal preferences, she explained that in tech, “We're not encouraged to pursue a more technical path, becoming instead generalists and taking responsibility for strategic parts of the business.”
There’s also the risk that gender biases can still affect career progression once in a tech or AI position. “We must not make assumptions that those creative roles are more appealing to women than they are to men,” warns Gjordeni.
The consequences of omitting women from the AI lifecycle are far-reaching. In recent years, global organisations and individuals have taken increasingly positive steps.
The United Nations and the World Economic Forum have both made a notable effort to start a global conversation about AI gender-based issues by developing initiatives that map out actionable strategies for businesses and political leaders to adopt.
Professionals like Peshtani and Gjordeni highlight the need for increased diversity and possibilities in tech. More significantly, they remind us that women haven’t gone anywhere.
“We can achieve greater results. The more diverse the workplace is, the better the results. We need to give equal opportunities to all people regardless of gender,” Peshtani concludes.