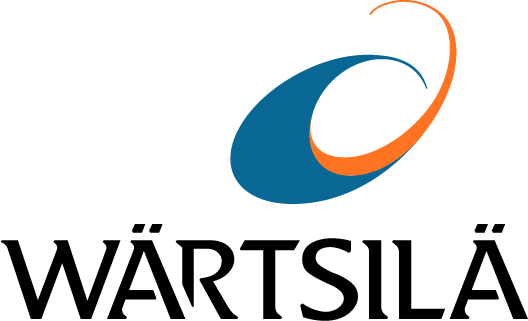
Innovative AI technology is transforming how energy is produced, stored, distributed, and consumed. The end result is not just convenience but a step towards a much greater cause - saving the planet.
Artificial Intelligence (AI) is everywhere, from virtual assistants helping you plan your day to how medical conditions are diagnosed. AI is also entering the energy industry and the results are quite promising.
Machine learning is the process when a computer system uses data to progressively improve the performance of a specific task and thereby become ‘intelligent’. Then, actions can be automated based on certain conditions or pattern recognition which can also anticipate future conditions. The energy industry uses this technology in a variety of applications, such as optimising production in oil and gas fields, generating compliance reports, and making predictions.
“Machine learning is being widely used to give a more accurate forecast of the demand and supply, like in case of wind generation,” explains Aidan O’Sullivan of University College London’s Bartlett School of Environment, Energy and Resources. “The UK’s national grid is deploying machine learning, which forecasts the carbon intensity of electricity 48 hours ahead.”
Renewable energy is becoming more important, but calm and cloudy days mean less solar and wind energy is generated. The gap is typically filled by traditional diesel or coal plants. However, when machine learning helps predict more accurately and manage these fluctuations in demand and supply, the whole system becomes more efficient.
Machine learning is also being used to lower energy consumption. O’Sullivan points to a fascinating use of reinforcement learning, where the system learns to make decisions by a reward function instead of historical data.
“Google recently handed over the management of their data centres to technology developed by Deepmind, which has reduced the energy consumption by some 40% through optimised performance,” he says.
Machine learning is also entering our homes with a demand-side response and IoT devices. These devices can reduce energy consumption at peak times, for instance, by automatically running washing machines late at night
Wärtsilä uses machine learning to manage energy storage systems and broader energy ecosystems. Their GEMS software platform interacts and integrates directly with the energy generation assets such as renewables, thermal, and also energy storage installations to operate and optimise the system’s performance.
“One good example of a project we have almost completed is on Graciosa, an island in the Azores,” says John Jung, former CEO of Greensmith Energy. “There, GEMS is managing an optimal mix of wind, solar, and diesel generators to minimise electricity costs and maximise sustainability by assessing local conditions and weather forecasts. It is effectively the brain behind improving electricity consumption pattern on an entire island that is transitioning from traditional generation to a hybrid plant with a cleaner grid. This island is small, but the technology could be scaled up for a much larger island, such as Australia.”
Energy storage is also becoming more and more important, and Jung points out that now even that segment of energy generation is programmable using the software. The GEMS platform has seven application families and 32 algorithms to match the custom needs and available resources for each project.
“GEMS can operate autonomously and automatically, based on either a rule-based engine or machine learning to act and function according to different or specific conditions sensed within the grid or integrated systems such as solar,” says Jung.
This technology is important to make the grid more resilient and secure – not just from intermittent supplies from renewables, but even from the hacking of vulnerable computer networks.
“When I started working with energy storage a decade ago, I knew that while batteries and other hardware were important, grid congestion was mainly a computing problem. Software, data and optimisation was the ‘killer app’ to change everything,” says Jung. “We have already begun that journey. AI as a viable approach to improve all asset-intensive industries will continue to flourish, except that it will do so at a geometrically faster rate.”
The use of machine learning can improve profitability in the industry while simultaneously making it more efficient, but O’Sullivan wants to draw attention to an even bigger issue: saving our planet.
“AI is potentially the greatest technological advance of our time and using it to solve the greatest challenge of our time – climate change – sounds poetic to me,” concludes O’Sullivan.